What is an Example of Data Intelligence?
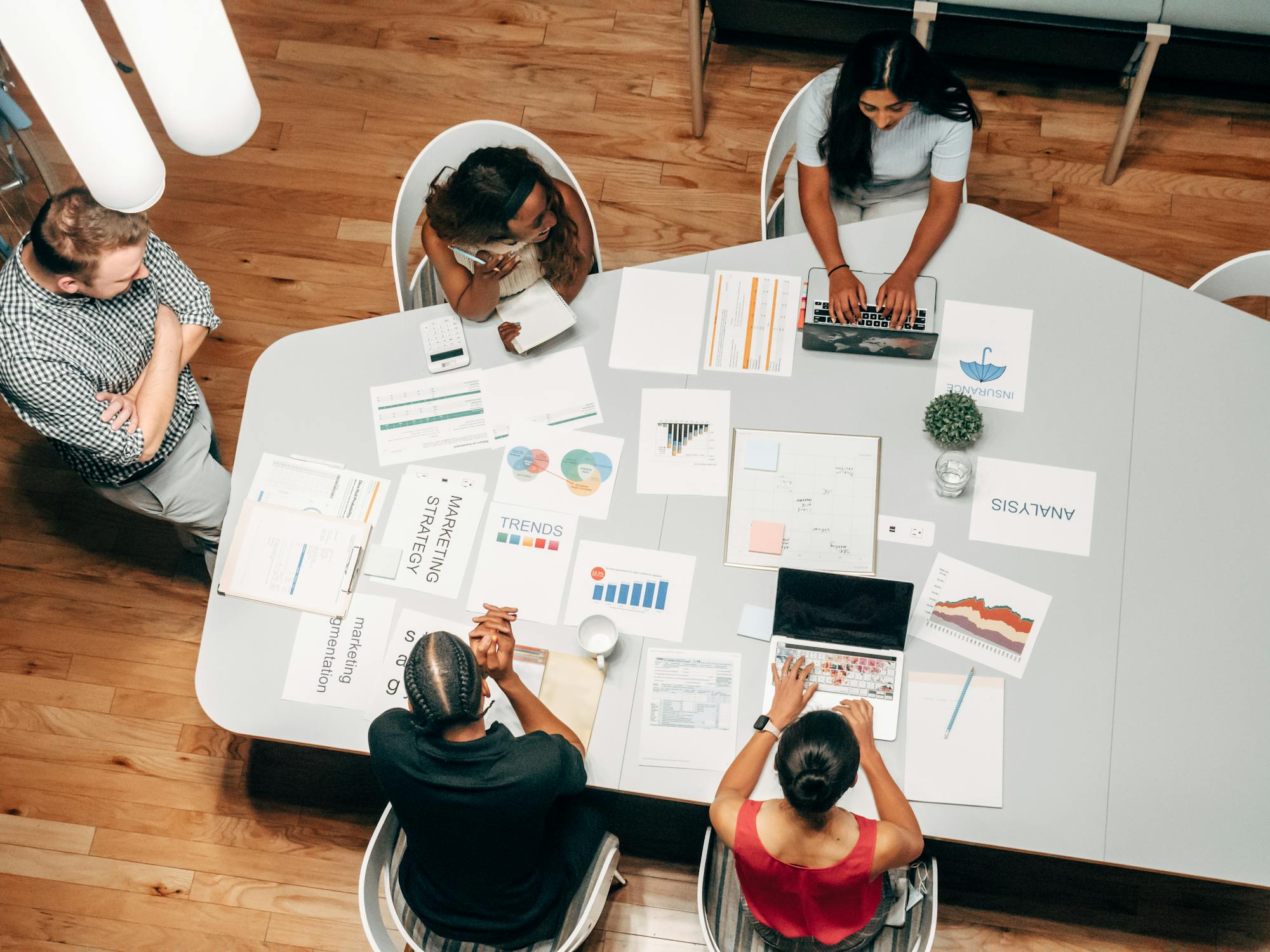
Data intelligence has transformed from a technical concept to a business necessity, enabling organizations to extract meaningful insights and drive strategic decisions. But what exactly does data intelligence look like in practice? This article explores real-world examples across industries that showcase how data intelligence is creating measurable value and competitive advantage.
Understanding Data Intelligence: Beyond Basic Analytics
Before diving into examples, it's important to understand what distinguishes data intelligence from more basic forms of data analysis. Where traditional analytics might tell you what happened, data intelligence answers the why, predicts what might happen next, and recommends actions to take.
Data intelligence combines multiple disciplines:
- Advanced analytics: Going beyond descriptive statistics to include predictive and prescriptive insights
- Artificial intelligence: Leveraging machine learning and other AI techniques to uncover patterns and automate decision processes
- Data integration: Combining diverse data sources to create a holistic view
- Business context: Aligning technical capabilities with domain expertise and specific business objectives
With this understanding, let's explore how data intelligence is creating transformative outcomes across different sectors.
Example 1: Predictive Maintenance in Manufacturing
One of the most compelling examples of data intelligence comes from the manufacturing sector, where predictive maintenance has revolutionized how companies manage equipment reliability and operational efficiency.
The Challenge
A global industrial equipment manufacturer was facing significant challenges with unplanned downtime. Each hour of downtime cost approximately $150,000 in lost production, and traditional maintenance approaches—either reactive (fix when broken) or preventive (scheduled maintenance regardless of condition)—were proving inefficient and costly.
The Data Intelligence Solution
The company implemented a comprehensive predictive maintenance system that integrated multiple data streams:
- Real-time sensor data from equipment (temperature, vibration, pressure, etc.)
- Maintenance records and equipment history
- Environmental and operational context data
- Manufacturer specifications and engineering knowledge
Advanced machine learning models were trained to detect subtle patterns that preceded equipment failures, often identifying issues days or weeks before they would cause a breakdown. The system continuously improved as it gathered more operational data and feedback on its predictions.
The Results
Key Outcomes:
- 92% reduction in unplanned downtime
- 30% decrease in overall maintenance costs
- 15% increase in equipment lifespan
- ROI of 545% over three years
What makes this a true data intelligence example rather than simply an analytics project is the combination of multiple data sources, advanced machine learning techniques, and the integration into operational workflows that created a self-improving system. The solution didn't just predict failures—it provided actionable recommendations for maintenance technicians and continuously refined its models based on outcomes.
Example 2: Dynamic Pricing Optimization in Retail
Retail competition has intensified dramatically in the digital age, with price sensitivity and comparison shopping just a click away. Leading retailers have embraced data intelligence to implement dynamic pricing strategies that maximize revenue while remaining competitive.
The Challenge
A multinational retailer with both online and physical stores was struggling to optimize pricing across 50,000+ SKUs. Manual pricing decisions were inconsistent, often reactive to competitors rather than strategic, and unable to account for complex factors like inventory levels, regional demand variations, and promotional effectiveness.
The Data Intelligence Solution
The retailer implemented an advanced dynamic pricing system that incorporated:
- Real-time competitor pricing data across both online and offline channels
- Historical sales data and price elasticity models
- Inventory levels and supply chain constraints
- Customer segmentation and behavior analysis
- Seasonal trends and event-based demand forecasts
The system used reinforcement learning algorithms to continuously test pricing strategies and learn from the results. It could automatically adjust prices within predefined guardrails, ensuring that pricing decisions aligned with overall brand positioning and margin requirements.
The Results
Key Outcomes:
- 7.2% increase in profit margin
- 3.5% increase in overall revenue
- 22% improvement in inventory turnover
- 18% reduction in price-matching claims
This example demonstrates how data intelligence goes beyond simple competitive price matching to create a strategic advantage. The system doesn't just react to market conditions but anticipates them, balancing immediate sales objectives with long-term customer value and brand positioning.
Example 3: Personalized Medicine in Healthcare
Healthcare is undergoing a profound transformation through data intelligence, with personalized medicine emerging as one of the most promising applications. By integrating diverse patient data with clinical knowledge and advanced analytics, healthcare providers can deliver more effective, individualized treatments.
The Challenge
A major healthcare system needed to improve outcomes for cancer patients while controlling treatment costs. Traditional one-size-fits-all treatment protocols often resulted in ineffective therapies, unnecessary side effects, and wasted resources. The specificity and complexity of cancer treatment made it an ideal candidate for data intelligence approaches.
The Data Intelligence Solution
The healthcare system implemented a comprehensive precision oncology platform that integrated:
- Genomic sequencing data to identify cancer-driving mutations
- Historical treatment outcomes from similar patients
- Electronic health records including comorbidities and medication histories
- Clinical trial data and emerging research
- Imaging analysis through computer vision algorithms
Machine learning models analyzed this integrated dataset to recommend personalized treatment plans, predict likely outcomes, and identify potential adverse effects. The system also flagged eligible clinical trials and emerging treatments that might benefit specific patient profiles.
The Results
Key Outcomes:
- 26% improvement in progression-free survival rates
- 32% reduction in serious adverse events
- 18% decrease in overall treatment costs
- 240% increase in clinical trial enrollment
This application of data intelligence is particularly powerful because it combines multiple complex data types—genomic, clinical, and research data—to create insights that would be impossible for human analysts to develop manually. The system augments physician expertise rather than replacing it, providing evidence-based recommendations that clinicians can incorporate into their decision-making.
Example 4: Fraud Detection in Financial Services
Financial institutions have been at the forefront of data intelligence adoption, particularly in the realm of fraud detection. Modern fraud prevention systems represent some of the most sophisticated applications of real-time data intelligence.
The Challenge
A global financial services provider was experiencing increasing fraud losses despite substantial investments in traditional rule-based detection systems. Fraudsters were continually adapting their techniques, and the existing systems generated too many false positives, creating customer friction and operational costs while still missing sophisticated fraud attempts.
The Data Intelligence Solution
The institution implemented an advanced fraud detection platform that leveraged multiple data intelligence capabilities:
- Real-time transaction monitoring with millisecond decision timelines
- Behavioral biometrics tracking typing patterns, device handling, and navigation
- Network analysis to identify connections between seemingly unrelated accounts
- Unsupervised anomaly detection to identify novel fraud patterns
- Adaptive models that evolve as new fraud techniques emerge
The system employed a multi-layered approach, combining rules, supervised machine learning models, unsupervised anomaly detection, and network analytics. This created a defense-in-depth strategy that could identify both known fraud patterns and emerging threats.
The Results
Key Outcomes:
- 63% reduction in fraud losses
- 42% decrease in false positive alerts
- 89% of fraud detected before losses occurred
- 18% improvement in customer satisfaction scores
This example demonstrates how data intelligence can solve seemingly contradictory objectives—reducing both fraud losses and false positives simultaneously. The combination of multiple analytical techniques and data sources creates a system that is both more accurate and more adaptable than traditional approaches.
Example 5: Supply Chain Optimization in Consumer Goods
Global supply chains have become increasingly complex, with vulnerabilities exposed by recent disruptions. Data intelligence has emerged as a critical tool for building more resilient, efficient supply networks.
The Challenge
A multinational consumer goods company with operations in 80+ countries was struggling with supply chain inefficiencies, including inventory imbalances, transportation costs, and vulnerability to disruptions. Traditional planning approaches relied on historical averages and simplistic forecasting, which failed to account for the complexity and variability of global operations.
The Data Intelligence Solution
The company implemented an end-to-end supply chain intelligence platform that incorporated:
- Multi-echelon inventory optimization using probabilistic demand forecasting
- Digital twin simulation of the entire supply network
- External data integration (weather, traffic, geopolitical events, etc.)
- Supplier risk assessment and alternative sourcing recommendations
- Scenario planning capabilities for potential disruptions
The system used a combination of machine learning for prediction and optimization algorithms for planning, creating a continuous adaptive planning process that could respond to changing conditions in near-real-time.
The Results
Key Outcomes:
- 22% reduction in inventory carrying costs
- 15% decrease in transportation expenses
- 97.8% service level achievement (up from 92.3%)
- 74% faster response to supply disruptions
This application of data intelligence demonstrates how advanced analytics can transform even the most complex operational challenges. By creating a digital representation of the entire supply network and continuously optimizing decisions based on real-time data, the company achieved seemingly contradictory goals—reducing costs while improving service levels and resilience.
Common Elements of Successful Data Intelligence Initiatives
Looking across these diverse examples, several common themes emerge that characterize successful data intelligence applications:
1. Integration of Multiple Data Sources
All of these examples leverage diverse data types—internal and external, structured and unstructured, historical and real-time—to create a comprehensive picture that would be impossible with siloed data.
2. Advanced Analytical Techniques
Simple descriptive or even predictive analytics aren't enough for true data intelligence. These examples employ sophisticated techniques like deep learning, reinforcement learning, network analysis, and simulation to extract deeper insights.
3. Continuous Learning and Adaptation
Static models quickly become outdated. The most effective data intelligence systems continuously learn from new data and feedback, constantly refining their predictions and recommendations.
4. Actionable Outputs
Data intelligence doesn't stop at insights—it delivers specific, actionable recommendations that can be directly integrated into business processes and decision workflows.
5. Human-AI Collaboration
Rather than automating decisions entirely, the most successful applications augment human expertise, providing decision support that combines the strengths of both human and artificial intelligence.
The Future of Data Intelligence
As these examples demonstrate, data intelligence is already delivering transformative value across industries. Looking ahead, several trends are poised to further amplify its impact:
Federated Learning and Privacy-Preserving Analytics
New techniques will enable organizations to gain insights from sensitive data without compromising privacy, creating opportunities for cross-organizational intelligence while maintaining regulatory compliance.
Explainable AI
As data intelligence becomes more embedded in critical decisions, the ability to understand and trust AI recommendations will become increasingly important. Advances in explainable AI will make complex models more transparent and interpretable.
Augmented Intelligence
The next generation of data intelligence will focus not just on automating decisions but on augmenting human capabilities, with AI and humans working as partners rather than replacements.
Edge Intelligence
As computing power at the edge increases, more intelligence will move from centralized data centers to local devices, enabling faster, more context-aware decisions without constant cloud connectivity.
Getting Started with Data Intelligence
Inspired by these examples but unsure how to begin? Here are some practical steps for organizations looking to harness the power of data intelligence:
- Start with clear business objectives, not technical capabilities. Identify specific challenges where better decisions would create measurable value.
- Assess your data readiness, including data quality, integration capabilities, and governance practices. Address foundational issues before moving to advanced analytics.
- Begin with focused, high-value use cases rather than attempting enterprise-wide transformation. Build momentum with early wins.
- Invest in both technology and talent. Data intelligence requires not just tools but also people with the skills to apply them effectively.
- Cultivate a data-driven culture that values evidence-based decision making and continuous learning.
Conclusion: The Transformative Power of Data Intelligence
The examples in this article illustrate how data intelligence is creating value across diverse industries and functions. From predictive maintenance and dynamic pricing to personalized medicine and fraud detection, organizations are leveraging the power of integrated data and advanced analytics to make better decisions, optimize operations, and create competitive advantage.
What sets these applications apart from traditional analytics is their ability to combine multiple data sources, employ sophisticated analytical techniques, continuously learn and adapt, and deliver actionable recommendations that drive measurable business outcomes.
As data volumes continue to grow and analytical capabilities advance, the potential for data intelligence will only increase. Organizations that embrace these capabilities today will be well-positioned to thrive in an increasingly data-driven future.
Team DataMinds Services
Data Intelligence Experts
The DataMinds team specializes in helping organizations leverage data intelligence to transform their businesses. Our experts bring decades of combined experience in data science, AI, business process management, and digital transformation.
More Articles
Ready to Harness the Power of Data Intelligence?
Contact our data experts today to discuss how we can help your organization transform data into actionable insights that drive measurable business value.
Contact Us Today