What is the Possible Outcome of Poor Data Quality?
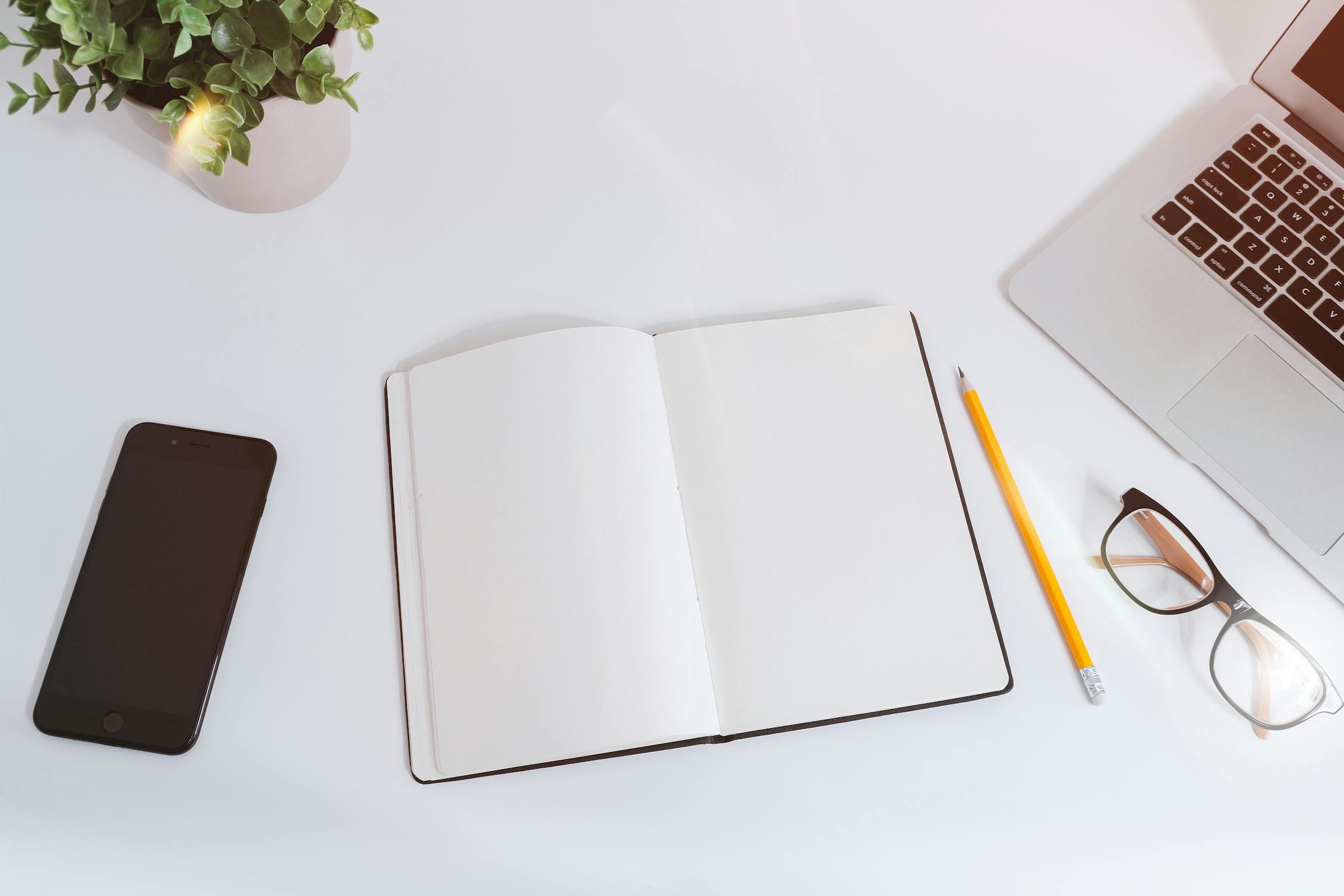
In today's data-driven world, organizations rely heavily on information to make strategic decisions, optimize operations, and understand customers. However, the value derived from data is directly tied to its quality. When data is poor—meaning it's inaccurate, incomplete, inconsistent, outdated, or invalid—the consequences can be severe and far-reaching, impacting nearly every aspect of the business.
The Ripple Effect of Bad Data
Poor data quality isn't just a minor inconvenience; it creates a cascade of negative outcomes. Let's explore some of the most significant possibilities:
1. Flawed Decision-Making
This is perhaps the most obvious outcome. If the data underpinning strategic choices, operational adjustments, or marketing campaigns is wrong, the decisions based on it are likely to be wrong too. This can lead to misallocation of resources, missed market opportunities, and failed initiatives. A low organizational Data IQ is often rooted in poor data quality.
2. Increased Operational Costs & Inefficiency
Bad data gums up the works. Employees waste valuable time identifying and correcting errors, reconciling conflicting information, or manually working around system limitations caused by data issues. This leads to:
- Increased manual effort and rework.
- Process delays and bottlenecks.
- Higher labor costs.
- Wasted resources (e.g., marketing materials sent to wrong addresses).
3. Financial Losses
The costs of poor data quality hit the bottom line directly and indirectly:
- Costs associated with operational inefficiencies (mentioned above).
- Missed revenue opportunities due to flawed market analysis or sales targeting.
- Fines and penalties for non-compliance due to inaccurate reporting (violating rules around Data Consistency or integrity).
- Costs of correcting large-scale data errors after they're discovered.
Studies consistently show that poor data quality costs companies significant percentages of their revenue annually.
4. Damaged Customer Relationships
Customers interact with data constantly, even if they don't realize it. Poor data quality can manifest as:
- Incorrect billing or shipping information.
- Irrelevant marketing messages or offers.
- Poor customer service experiences due to agents having wrong information.
- Difficulty accessing services or accounts.
These experiences erode customer trust and loyalty, potentially leading to churn.
5. Reduced Productivity & Employee Morale
Constantly battling bad data is frustrating for employees. Spending time cleaning up messes, dealing with confused customers, or lacking confidence in reports can lead to burnout, reduced job satisfaction, and lower overall productivity.
6. Compliance and Legal Risks
Many industries face strict regulatory requirements regarding data accuracy and reporting (e.g., finance, healthcare). Poor data quality can lead to non-compliance, resulting in hefty fines, legal action, and damage to the company's standing. Issues often stem from a lack of Data Reliability or integrity.
7. Failure of Analytics, BI, and AI Initiatives
Advanced analytics, business intelligence dashboards, and AI/machine learning models are only as good as the data they are fed. "Garbage in, garbage out" holds true. Poor data quality leads to:
- Inaccurate reports and dashboards.
- Biased or incorrect analytical models.
- Failed AI implementations.
- Wasted investment in data science tools and talent.
8. Erosion of Trust and Data Culture
When users frequently encounter errors or inconsistencies, they lose trust in the data and the systems providing it. This undermines efforts to foster a data-driven culture, as people revert to intuition or guesswork rather than relying on information they perceive as unreliable. Effectively discussing data becomes impossible if no one trusts it.
9. Reputational Damage
Significant data errors, especially those affecting customers or compliance reporting, can become public knowledge, leading to negative press, loss of stakeholder confidence, and long-term damage to the organization's reputation.
Conclusion: A Cost Too High to Ignore
The potential outcomes of poor data quality are not minor inconveniences; they are significant business risks that can cripple decision-making, inflate costs, alienate customers, and undermine strategic initiatives. Investing in data quality management—including governance, profiling, cleansing, and monitoring—is not just a technical necessity but a fundamental business imperative for survival and success in the modern economy. Ignoring data quality is simply too costly.
Is poor data quality hindering your organization? DataMinds.Services can help you diagnose issues and implement solutions to improve data accuracy, consistency, and overall quality.
Team DataMinds Services
Data Intelligence Experts
The DataMinds team specializes in helping organizations leverage data intelligence to transform their businesses. Our experts bring decades of combined experience in data science, AI, business process management, and digital transformation.
More Articles
Are Data Quality Issues Costing Your Business?
Don't let poor data undermine your success. Contact DataMinds Services to assess your data quality and implement strategies for lasting improvement.
Assess Your Data Quality